Before we start
Two of my previous post might be helpful in getting a general understanding of the top solutions of this competition. Please feel free to check them out.
- Knowledge Distillation clearly explained
- Common Multilingual Language Modeling methods (M-Bert, LASER, MultiFiT, XLM)
Jigsaw Multilingual Toxic Comment Classification
Use TPUs to identify toxicity comments across multiple languages.
Overview of the competition
Jigsaw Multilingual Toxic Comment Classification is the 3rd annual competition organized by the Jigsaw team. It follows Toxic Comment Classification Challenge, the original 2018 competition, and Jigsaw Unintended Bias in Toxicity Classification, which required the competitors to consider biased ML predictions in their new models. This year, the goal is to use English only training data to run toxicity predictions on foreign languages (tr, ru, it, fr, pt, es).
Kagglers are predicting the probability that a comment is toxic. A toxic comment would receive a 1.0
. A benign, non-toxic comment would receive a 0.0
. In the test set, all comments are classified as either a 1.0
or a 0.0
. The whole test set was visible in this competition.
Data
jigsaw-toxic-comment-train.csv
: from Jigsaw Toxic Comment Classification Challenge (2018).
0.0 |
1.0 |
total | |
---|---|---|---|
count | 202165 | 21384 | 223549 |
jigsaw-unintended-bias-train.csv
: from Jigsaw Unintended Bias in Toxicity Classification (2019)
0.0 |
1.0 |
total | |
---|---|---|---|
count | 1789968 | 112226 | 1902194 |
validation.csv
: comments from Wikipedia talk pages in different non-English languagestest.csv
: comments from Wikipedia talk pages in different non-English languages
Here is the the value counts in valid data:
0.0 |
1.0 |
total | |
---|---|---|---|
es | 2078 | 422 | 2500 |
it | 2012 | 488 | 2500 |
tr | 2680 | 320 | 3000 |
Here is the the value counts in test data:
As you can see, the test set comments contains 6 non-English languages (tr, ru, it, fr, pt, es) and the validation set contains only three non-English comments (es, it, tr).
1st place solution
Ensembling to mitigate Transformer training variability
Since the performance of Transformer models is impacted heavily by initialization and data order, they went with an iterative blending approach, refining the test set predictions across submissions with a weighted average of the previous best submission and the current model’s predictions. They began with a simple average, and gradually increased the weight of the previous best submission.
Note: The predictions are an exponential moving average of all past model predictions and the current model’s prediction.
Pseudo-Labeling
They observed a performance improvement when they used test-set predictions as training data - the intuition being that it helps models learn the test set distribution. Using all test-set predictions as soft-labels worked better than any other version of pseudo-labelling (e.g., hard labels, confidence thresholded PLs, etc). Towards the end of the competition, we discovered a minor but material boost in LB when we upsampled the PLs.
Multilingual XLM-Roberta models
As with most teams, they began with a vanilla XLM-Roberta model, incorporating translations of the 2018 dataset in the 6 test-set languages as training data. They used a vanilla classification head on the [CLS] token of the last layer with the Adam optimizer and binary cross entropy loss function, and finetuned the entire model with a low learning rate. Given Transformer models having several hundred million trainable weights put to the relatively simple task of making a binary prediction, they didn’t spend too much time on hyper-parameter optimization, architectural tweaks, or pre-processing.
Train foreign language monolingual Transformer
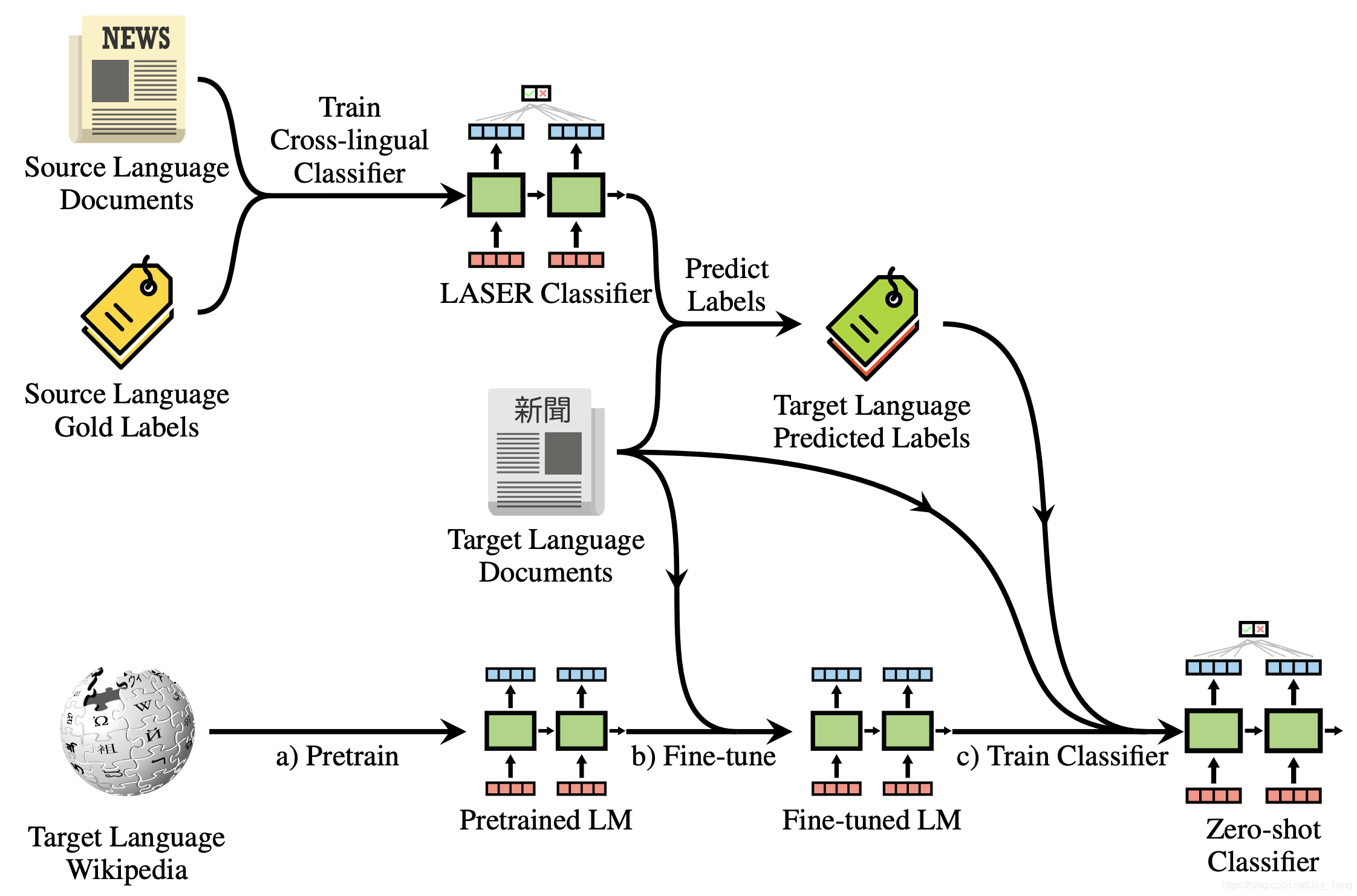
Inspired by the MultiFiT paper, they observed a dramatic performance boost when they used pretrained foreign language monolingual Transformer models from HuggingFace for the test-set languages(e.g., Camembert for french samples, Rubert for russian, BerTurk for turkish, BETO for spanish, etc).
They finetuned models for each of the 6 languages:
- Combining translations of the 2018 Toxic Comments together with pseudo-labels for samples from the test set and hard labels for samples from the val set in that specific language. These pseudo-labels initially come from the XLM-R multilingual models, then they are continuously refined by these monolingual transformer models.
Note: A training run would for example have 10K test set samples + 70K subsampled 2018 translations samples + 2.5K val samples. These models are quite good at few-shot learning so <100K is sufficient to learn.
- Training the corresponding monolingual model, predicting the same samples then blending it back with the “main branch” of all predictions. It was synergistic in that training cross-lingual model -> monolingual models -> cross-lingual model, etc. lead to continual performance improvements.
For each model run, they would reload weight initalizations from the pretrained models to prevent overfitting. In other words, the continuing improvements of the predictions were being driven by refinements in the pseudo-labels we were providing to the models as training data.
For a given monolingual model, predicting only test-set samples in that language worked best. Translating test-set samples in other languages to the model’s language and predicting them worsened performance.
Finetuning pre-trained foreign language monolingual FastText models
After they exhausted the HuggingFace monolingual model library, they trained monolingual FastText Bidirectional GRU models on 2018 Toxic Comments, using pretrained embeddings for the test-set languages, to continue refining the test set predictions (albeit with a lower weight when combined with the main branch of predictions) and saw a small but meaningful performance boost.
Post-processing
Intuition:
They consider the trend of subsequent submissions of a specific language (e.g. Russian) for each example in the test dataset. If the trend of that example is positive, they nudge the example further in the positive direction and vice versa.
They measure the trend by taking the differences of all subsequent submissions for the specific language and averaging those differences. The nudge that they give to the new submission is based on a predefined weight, typically a weight of 1 or 1.5.
Pseudo-code
Given the following:
weight = predefined weight (typically 1 or 1.5)
pred_best = current best predictions on LB
diff_avg = average of differences of consecutive subs (trend)
Then for each example in test of the specific language (e.g. Turkish):
if diff_avg < 0: # negative trend
pred_new = (1+weight*diff_avg)*pred_best # nudge downwards
else: # positive trend
pred_new = (1-weight*diff_avg)*pred_best + diff_avg # nudge upwards
4th place solution special
Speeding up training
-
One way is to downsample negative samples to get a more balanced dataset and a smaller dataset at the same time. If you only use as many negative as positive then dataset is reduced 5x roughly, same for time to run one epoch.
-
Another speedup comes from padding by batch. The main idea is to limit the amount of padding to what is necessary for the current batch. Inputs are padded to the length of the longest input in the batch rather than a fixed length. It has been used in previous competitions and it accelerates training significantly. They refined the idea by sorting the samples by their length so that the samples in a given batch have similar length. This reduces even further the need for padding. If all inputs in the batch have the same length then there is no padding at all. Given samples are sorted, they cannot be shuffled in the training mode. They rather shuffled batches. This yields an extra 2x speedup compared to the original padding by batch.
To learn how to implement padding by batch, read my previous summary on Tweeter Sentiment Extraction section sequence bucketing (dynamic padding).
Training strategy
One main ingredient for their good result is a stepwise finetuning towards single language models for tr, it and es. Below is the illustration of their model architecture:
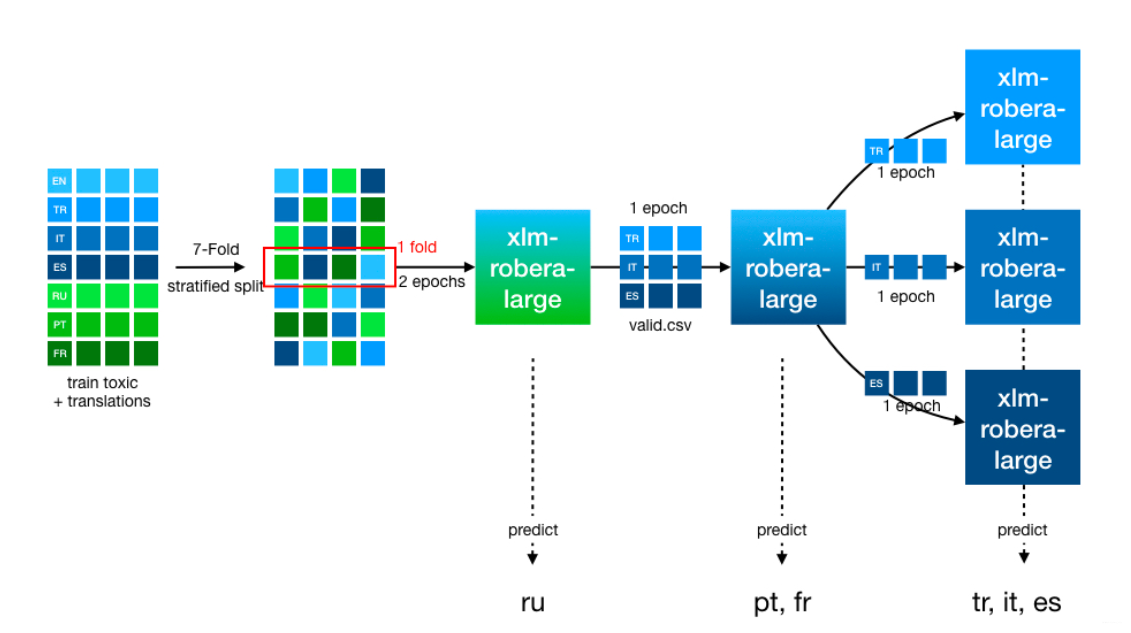
As input data, they used the english 2018 Toxic Comments and its six translations available as public datasets which combined are roughly 1.4M comments. Training a model directly on the combined dataset is not optimal, as each comment appears 7x in each epoch (although in different languages) and the model overfits on that. So they divided the combined dataset into 7 stratified parts, where each part contains a comment only once. For each fold they then fine-tuned a transformer in a 3 step manner:
- Step 1: finetune to all 7 languages for 2 epochs
- Step 2: finetune only to the full valid.csv which only has tr, it and es
- Step 3: 3x finetune to each language in valid resulting in 3 models
We then use the step1 model for predicting ru, the step2 model for predicting pt and fr and the respective step3 models for tr, it and es. Using the step2 model for pt and fr gave a significant boost compared to using step1 model for those due. Most likely due to the language similarity between it, es, fr and pt.
Post-processing
The competition metric is sensitive to the global rank of all predictions and not language specific. So they took care of the languages having a correct relation to each other, by shifting the predictions of each language individually.
test_df.loc[test_df["lang"] == "es", "toxic"] *= 1.06
test_df.loc[test_df["lang"] == "fr", "toxic"] *= 1.04
test_df.loc[test_df["lang"] == "it", "toxic"] *= 0.97
test_df.loc[test_df["lang"] == "pt", "toxic"] *= 0.96
test_df.loc[test_df["lang"] == "tr", "toxic"] *= 0.98
I tried out this post-processing and it boosted my rank from 64th to top 30.
Commons in top solutions
I read through top 10 solutions and indeed they are roughtly the same. Unlike in Tweet Sentiment Extraction where every top solution has its own novel and fancy model structures, top solutions in this competition mostly share the following ideas:
-
Pseudo-Labeling (using soft labels).
-
Use monolingual Transformer models.
-
Two stage training.
-
Post-processing (each team have has different pp strategy).
Two stage training
This is the last common ideas that I haven’t explained. The main idea is the following.
-
One stage training means that we always train the same data in training. This policy always get the lower scores.
-
Two stage training means that we will have two different data for training. e.g., firstly train on Toxic Comment Classification dataset (+ Unintended Bias in Toxicity dataset). After that train on 8k validation data. This policy always get the higher scores.
A checklist of contemporary NLP classification techniques
1st place author shared a checklist of contemporary NLP classification techniques. The followings are a selection of what didn’t work for them in this competition, but I still believe these ideas are worth trying in any competition or project.
- Document-level embedders (e.g., LASER)
- Further MLM pretraining of Transformer models using task data
- Alternative ensembling mechanisms (rank-averaging, stochastic weight averaging)
- Alternative loss functions (e.g., focal loss, histogram loss)
- Alternative pooling mechanisms for the classification head (e.g., max-pool CNN across tokens, using multiple hidden layers etc.)
- Non-FastText pretrained embeddings (e.g., Flair, glove, bpem)
- Freeze-finetuning for the Transformer models
- Regularization (e.g., multisample dropout, input mixup, manifold mixup, sentencepiece-dropout)
- Backtranslation as data augmentation
- English translations as train/test-time augmentation
- Self-distilling to relabel the 2018 data
- Adversarial training by perturbing the embeddings layer using FGM
- Multi-task learning
- Temperature scaling on pseudo-labels
- Semi-supervised learning using the test data
- Composing two models into an encoder-decoder model
- Use of translation focused pretrained models (e.g., mBart)
Reference:
- 1st place solution: https://www.kaggle.com/c/jigsaw-multilingual-toxic-comment-classification/discussion/160862, https://www.kaggle.com/c/jigsaw-multilingual-toxic-comment-classification/discussion/160986
- 4th place solution: https://www.kaggle.com/c/jigsaw-multilingual-toxic-comment-classification/discussion/160980
- 6th place solution: https://www.kaggle.com/c/jigsaw-multilingual-toxic-comment-classification/discussion/161095
- 11st place solution: https://www.kaggle.com/c/jigsaw-multilingual-toxic-comment-classification/discussion/160961
- Jigsaw Multilingual: Quick EDA & TPU Modeling: https://www.kaggle.com/ipythonx/jigsaw-multilingual-quick-eda-tpu-modeling
- Jigsaw Train Multilingual Coments (Google API) For multilingual models training: https://www.kaggle.com/miklgr500/jigsaw-train-multilingual-coments-google-api
The followings are monolingual language models in huggingface communities mentioned by the 6th place solution:
- es:
- fr:
- tr:
- (128k vocabulary) https://huggingface.co/dbmdz/bert-base-turkish-128k-uncased
- (128k vocabulary) https://huggingface.co/dbmdz/bert-base-turkish-128k-cased#
- (32k vocabulary) https://huggingface.co/dbmdz/bert-base-turkish-uncased#
- (32k vocabulary) https://huggingface.co/dbmdz/bert-base-turkish-cased#
- it
- pt
- ru