Authors: Kevin Clark, Urvashi Khandelwal, Omer Levy, Christopher D. Manning
Paper reference: https://arxiv.org/pdf/1906.04341.pdf
Before we start
In this post, I mainly focus on the conclusions the authors reach in the paper, and I think these conclusions are worth sharing.
In this paper, the authors study the attention maps of a pre-trained BERT model. Their analysis focuses on the 144 attention heads in BERT.
Surface-Level Patterns in Attention
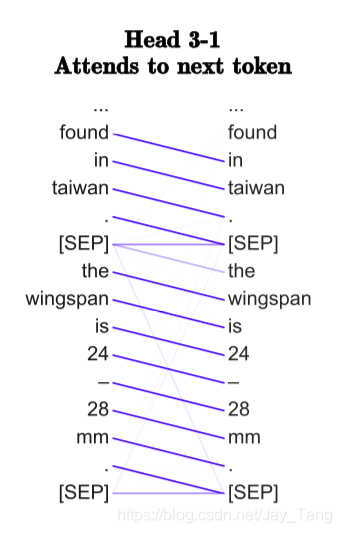
- There are heads that specialize to attending heavily on the next or previous token, especially in earlier layers of the network.
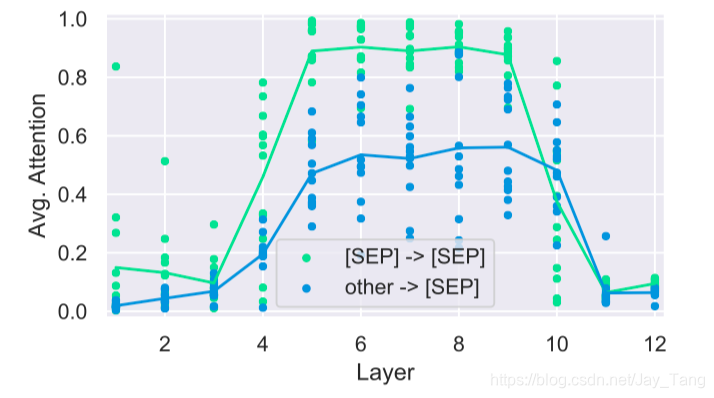
-
A substantial amount of BERT’s attention focuses on a few tokens. For example, over half of BERT’s attention in layers 6-10 focuses on [SEP]. One possible explanation is that [SEP] is used to aggregate segment-level information which can then be read by other heads.
However, if this explanation were true, they would expect attention heads processing [SEP] to attend broadly over the whole segment to build up these representations. However, they instead almost entirely (more than 90%) attend to themselves and the other [SEP] token.
They speculate that attention over these special tokens might be used as a sort of “no-op” when the attention head’s function is not applicable.
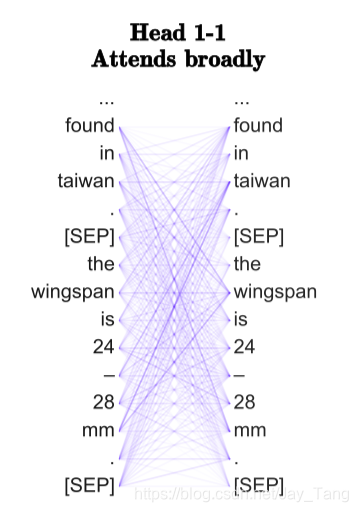
-
Some attention heads, especially in lower layers, have very broad attention. The output of these heads is roughly a bag-of-vectors representation of the sentence.
-
They also measured entropies for all attention heads from only the [CLS] token. The last layer has a high entropy from [CLS], indicating very broad attention. This finding makes sense given that the representation for the [CLS] token is used as input for the “next sen- tence prediction” task during pre-training, so it attends broadly to aggregate a representation for the whole input in the last layer.
Probing Individual Attention Heads
-
There is no single attention head that does well at syntax “overall”.
-
They do find that certain attention heads specialize to specific dependency relations, sometimes achieving high accuracy.
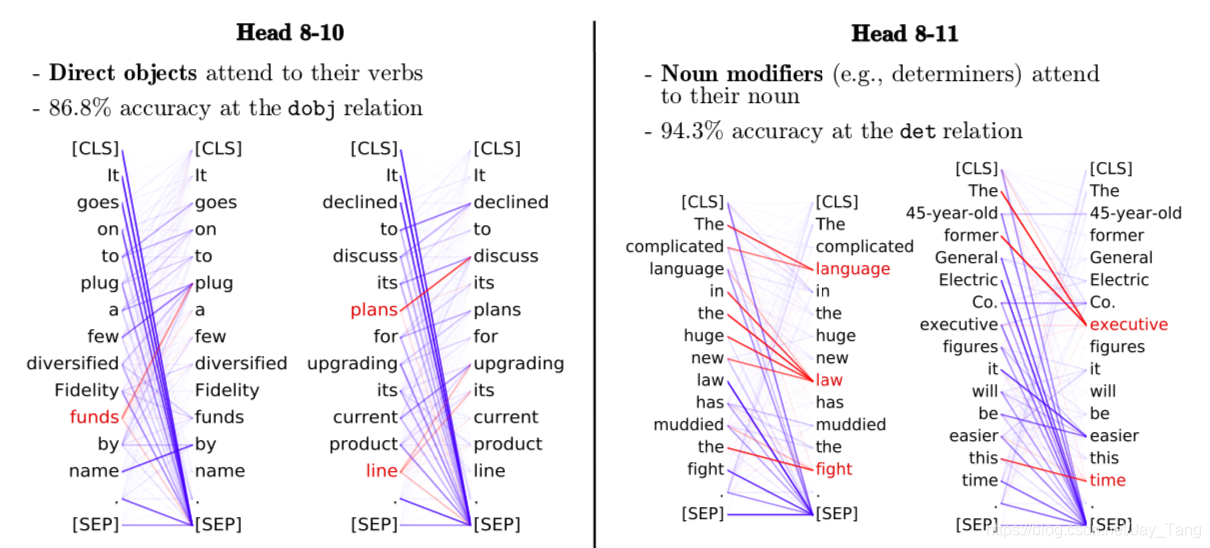
-
Despite not being explicitly trained on these tasks, BERT’s attention heads perform remarkably well, illustrating how syntax-sensitive behavior can emerge from self-supervised training alone.
-
While the similarity between machine-learned attention weights and human-defined syntactic relations are striking, they note these are relations for which attention heads do particularly well on. They would not say individual attention heads capture dependency structure as a whole.
Probing Attention Head Combinations
The probing classifiers are basically graph-based dependency parsers. Given an input word, the classifier produces a probability distribution over other words in the sentence indicating how likely each other word is to be the syntactic head of the current one.
-
Their results from probing both individual and combinations of attention heads suggest that BERT learns some aspects syntax purely as a by-product of self-supervised training.
-
A growing body of work indicating that indirect supervision from rich pre-training tasks like language modeling can also produce models sensitive to language’s hierarchical structure.
Clustering Attention Heads
- Heads within the same layer are often fairly close to each other, meaning that heads within the layer have similar attention distributions. This finding is a bit surprising given that Tu et al. (2018) show that encouraging attention heads to have different behaviors can improve Transformer performance at machine translation.
Computing the distances between all pairs of attention heads. Formally, they measure the distance between two heads $\mathrm{H}_{i}$ and $\mathrm{H}_{j}$ as:
$$ \sum_{\text {token } \in \text { data }} J S\left(\mathrm{H}{i}(\text { token }), \mathrm{H}{j}(\text { token })\right) $$
Where $J S$ is the Jensen-Shannon Divergence between attention distributions. Using these distances, they visualize the attention heads by applying multidimensional scaling to embed each head in two dimensions such that the Euclidean distance between embeddings reflects the Jensen-Shannon distance between the corresponding heads as closely as possible.
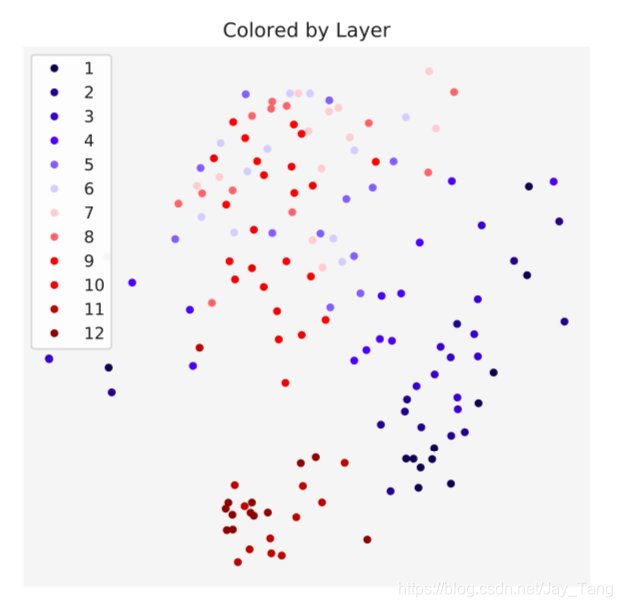
-
Heads within the same layer are often fairly close to each other, meaning that heads within the layer have similar attention distributions. This finding is a bit surprising given that Tu et al. (2018) show that encouraging attention heads to have different behaviors can improve Transformer performance at machine translation.
-
Many attention heads can be pruned away without substantially hurting model performance. Interestingly, the important attention heads that remain after pruning tend to be ones with identified behaviors.
Reference:
- What Does BERT Look At? An Analysis of BERT’s Attention: https://arxiv.org/pdf/1906.04341.pdf